Discover the profound impact of citizen science in strengthening machine learning through crowdsourced data mining. Explore real-world use cases and the collaborative efforts driving cutting-edge research at the intersection of these fields. Delve into the fascinating world where citizen science empowers machine learning, and witness the transformative potential of this unique approach.
Challenge
The first challenge tackled in this use case is the explosion in the volume and complexity of scientific data. Many scientific disciplines have begun a new data-rich era of discovery, from genomics to astronomical imaging to social sciences working with the firehose of data from social networks. But the data avalanche is so fast, so large and so complex that it often presents serious challenges for computing. Machine learning / artificial intelligence can alleviate these challenges, but this is usually contingent on having sufficient labelled data sets to act as training sets for machine learning. Unsupervised machine learning technologies are often insufficient to the task. Some open science data mining problems are at present completely intractable even for machine learning, particularly where a human assessment is intrinsic to the problem at hand, and even the most sophisticated AI technologies are unable to respond to a classification problem with, in effect, “Seeing the data, this is the wrong question, because the data are telling us something unexpected”.
A second challenge is that the science-inclined public is by far the largest, but often most overlooked, set of stakeholders in open science. A central vision of the European Open Science Cloud (EOSC) is to make scientific data FAIR, that is Findable, Accessible, Interoperable and Reusable. Implicit in this vision is that FAIR data should also be useful, but this is far from being guaranteed, especially given its inter- and multi-disciplinary remit. There are many examples of errors in FAIR data use by researchers outside their direct specialism. The farther from one’s subject specialism, the more curated one’s interaction with data needs to be. This goes beyond the simplest expressions of metadata, and may require specialist training. The science-inclined public is at the farthest limit, and needs the most support in their interaction with FAIR data.
Solution
Humans are still often much better than machine learning at many classification tasks. This has led to a new way of doing science: crowdsourcing, with the help of citizen science volunteers. This gives members of the public a genuine and valuable participation in scientific discovery, and there is a huge public appetite for taking part. We therefore aim to involve society at large with open science much more directly, through mass participation experiments.
To integrate this crowdsourced data mining into EOSC, we have been working closely with the ESFRIs Science Analysis Platform (ESAP), since it is designed with multi-disciplinary working in mind, it supports interactive analyses and deep integration with the data lake and with batch computing. ESAP users are currently able to query the back-end database of the Zooniverse citizen science platform, load the results into their ESAP Shopping Basket, and then send them to analysis services for further processing.
Our solution starts with professional scientists working already on (for example) ESAP, using data from its open science data lake. On that platform they are working with the data from their citizen science experiments and ultimately managing the citizen science projects themselves throughout the project lifecycle. Volunteers themselves then have the ability to link out of the citizen science projects into the professional tools, armed with the new knowledge that they have acquired as part of the citizen science project that gives them the context of the scientific data.
In summary, we have the science platform managing the data and citizen scientists generating the data, while the professional scientists would like of course to accelerate the classifications of their data with the help of machine learning. Therefore, the intention is that a professional scientist would work within a science platform, training machine learning algorithms based on the truth sets from the citizen science data. Having done that, one can use the machines to classify the most straightforward and unambiguous items. Then the project will be able to refocus the human effort on the difficult edge cases that are the most sensible use of human effort. Therefore, one achieves a virtuous circle between human and machine classification.
Our overarching vision is to improve access to data and tools through these citizen science crowd-sourcing experiments. Contextual educational and training resources are embedded into the volunteer workflows. This allows non-specialist volunteers to gain enough subject specialist knowledge for more comprehensive explorations of the data, and indeed on many such projects there are explicit links to external professional tools for this deeper engagement.
Crucial to this vision is our definition of citizen science as scientifically-driven crowdsourced data mining and data collection, which happens to involve non-specialist volunteers. In particular, note what citizen science is not: outreach. Citizen science can of course help with outreach, but that is not what it is for. It is perhaps more appropriate to think of crowd-sourced data mining as the application of a biological computer. As with any other scientific tool or facility, such as a beamline or a spectrometer, there are science questions that are particularly well suited to the tool.
Impact
Citizen science has the advantage of involving a much larger and more diverse scientific user community with EOSC, and indeed the citizen community is one of the strategic priorities of the EOSC Strategic Research and Innovation Agenda. The capability to access Zooniverse crowd-sourced data mining products from within the ESCAPE Science Analysis Platform includes projects within the cross-cluster scientific domains SSHOC, EOSC-Life, ENVRI-FAIR, PANOSC, and ESCAPE, with over 100 active projects and around 2 million volunteers.
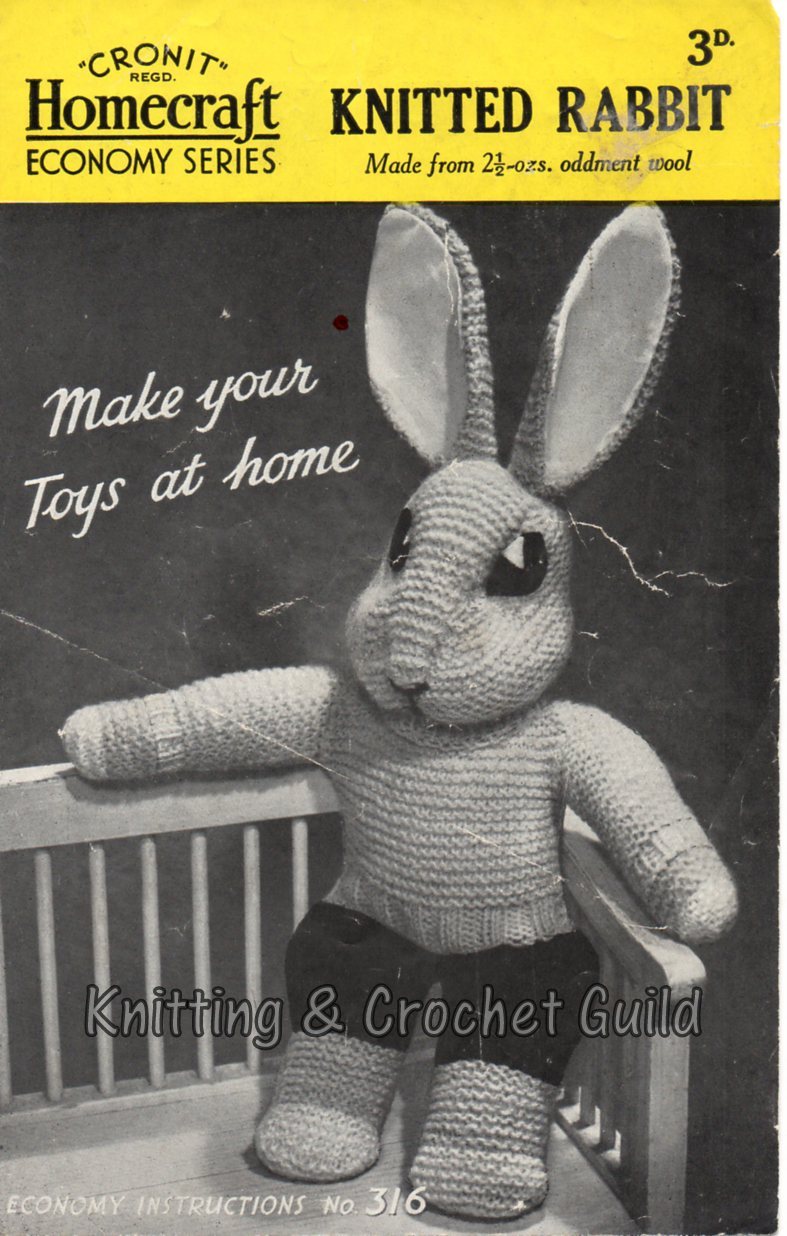
With the help of the EOSC-Future and ESCAPE projects, we have already built many citizen science demonstrators, including:
- Knitting patterns citizen science project which aims to discover more about how knitting and knitwear developed in Britain during the twentieth century, by carefully characterising information in knitting pattern leaflets and magazines, to yield knowledge of how versions of femininity connected to fashion and consumption, and how they changed over time (please note that this is currently in beta testing. by the Zooniverse community and therefore subject to change)
- African Indigenous Knowledge which aims to capture, document and share African indigenous food system knowledge for promoting sustainable nutritious food production, processing and consumption in Africa (please note this is currently still in development, so the currently visible volunteer workflows may change).
- Galaxy Zoo: Clump Scout which aimed to find the clumps in galaxies where stars are being born, and work out why these clumps were much more common in the distant past. This project is now completed, with 14,285 volunteers contributing over 1.7 million classifications, which have now been used for training machine learning.
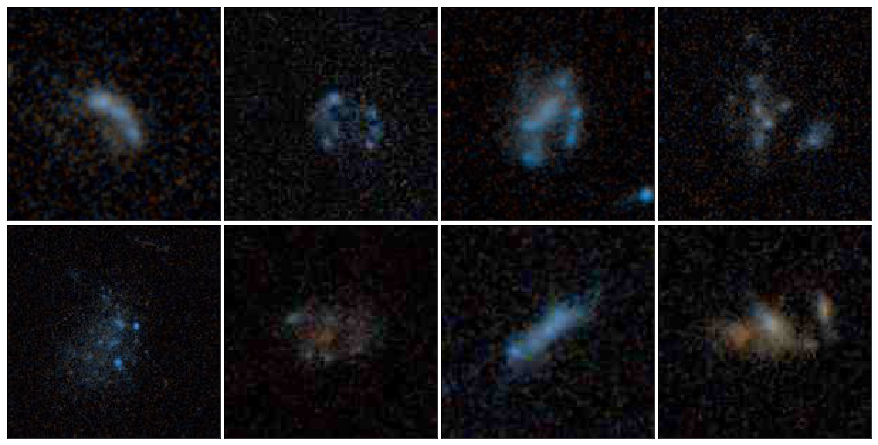
Images of distant clumpy galaxies, taken by the Hubble Space Telescope. The blue clumps are regions of vigorous star formation that can be identified by volunteers.
Ultimately, our vision is for the community to access clear exemplars of planning, creating and managing crowd-sourced data mining in EOSC, implementing machine learning in real time, across a wide range of scientific domains, which they can use as templates to deploy with ease. Through this, our vision is to increase the size of the community making real scientific engagement with EOSC by orders of magnitude, solving the difficult problem of usefulness of FAIR data by giving non-specialists a carefully curated and educationally supportive experience of EOSC.
Prof. Stephen Serjeant
For practical software for running Zooniverse projects:
For more information on the Zooniverse platform, visit the official website.
For information about how Zooniverse activities are integrated into the European Open Science Cloud, visit this link.
Browse the different disciplinary sections in Zooniverse via the filters below: